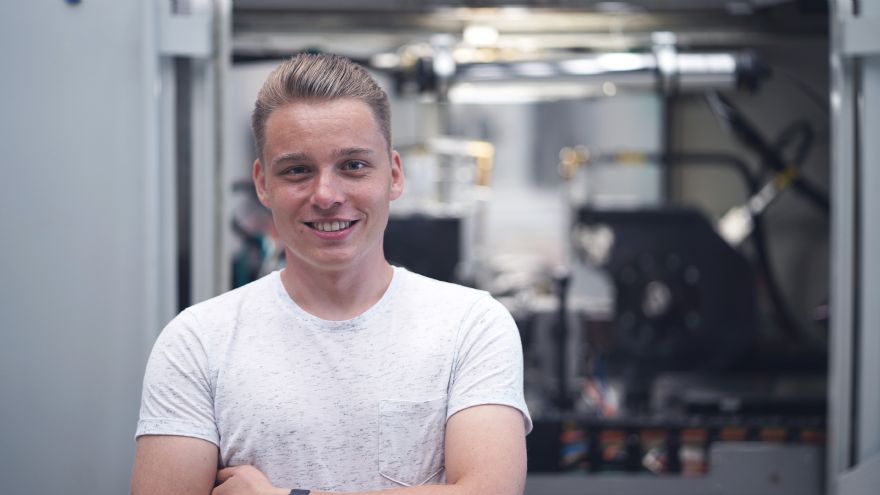
Fritz Studer AG has awarded its research prize, the ‘Fritz Studer Award’, for the seventh time. Applicants from several European countries submitted their work and Dr Emil Sauter won the award and the prize of CHF10,000.
Dr Frank Fiebelkorn, head of research and technology at Fritz Studer AG, said: "The Fritz Studer Award is aimed at graduates from European universities and technical colleges and we were looking for creative ideas and solutions for the machine tool industry. The objectives of the research prize are, on the one hand, to boost innovation in the machine tool industry with feasible solutions, and on the other hand, to promote young technical and scientific talents.”
The focus on submissions have to be on specific topics: innovative machine concepts or components for machine tools used in precision machining; alternative materials in the machine tool industry; simulation models for the dynamic and thermal behavior of machine tools; control and sensor concepts for machine tools; and new or evolved manufacturing techniques, especially in precision finishing processes like grinding or hard turning.
Numerous undergraduate/master’s theses and dissertations were submitted to Studer. The submissions were evaluated by a panel of judges that included Professor Konrad Wegener, Inspire Institute for Machine Tools and Manufacturing at ETH Zurich, Dr Hans-Werner Hoffmeister, former head of the Manufacturing Technology department at the Institute for Machine Tools and Manufacturing Technology of the TU Braunschweig, and Dr Frank Fiebelkorn, head of research and technology at Fritz Studer AG.
Criteria for evaluating the works included the feasibility of the findings in the mechanical engineering industry, the degree of innovation and quality of the research idea, scientific content, form, and the accuracy of statements, and the results and findings.
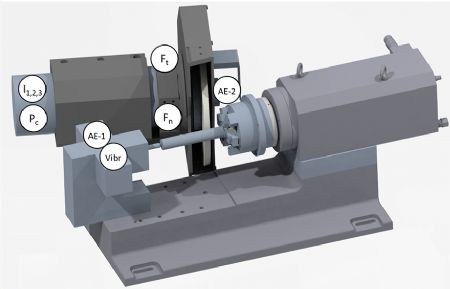
The
Fritz Studer Award 2023 was presented to Dr Emil Sauter from the Institute for Manufacturing Technology and Machine Tools at ETH Zurich earlier this month. He impressed the entire jury with his thesis
Detection and avoidance of thermal damage for high-performance metal grinding processes using hybrid machine learning models.
His work deals with the development of an innovative condition monitoring system for external cylindrical grinding with metal-bonded CBN tools, which recognises thermal damage in situ and predicts the remaining useful life of grinding tools with relative accuracy. Process parameters such as structure-borne noise, spindle current, force characteristics, and the use of time-frequency transformations generate process features of a grinding process to detect different stages of thermal damage to the workpiece with high accuracy. The remaining tool life can also be estimated.
This work with its many industry-oriented practical tests proves that machine learning methods can lead to higher productivity and improved component quality in grinding technology.